Predictive value of an MRI-based deep learning model for lymphovascular invasion status in lymph node-negative invasive breast cancer
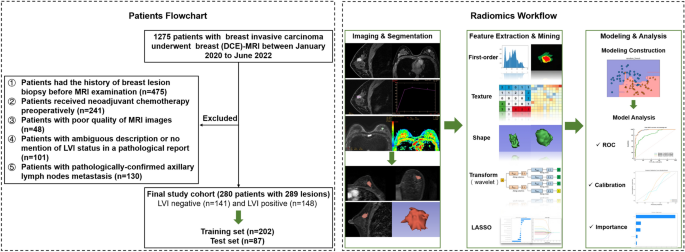
Patients
This retrospective study was approved by the Medical Ethics Committee of Tianjin Cancer Hospital, which waived the requirement for informed consent and conducted all examinations in accordance with relevant guidelines. We recruited a total of 1275 consecutive patients diagnosed with invasive breast cancer between January 2020 and June 2022. The exclusion criteria are listed in Appendix E1. Ultimately, 280 patients with 289 lesions were included. These patients were randomly grouped into training datasets (n = 202) and validation datasets (n = 87) at a ratio of 7:3. The overall study design is shown in Fig. 1.
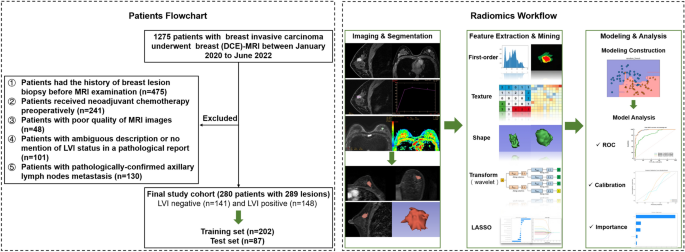
The general workflow of the study. Patient recruitment and random grouping are shown in the left dotted box. The radiomics workflow is shown in the right dotted box.
Image capture protocol
MRI examinations were performed on patients in the prone position using a 1.5 T scanner (Signa HDxt, GE Healthcare, USA) and a 3.0 T scanner (Signa Pioneer and Discovery 750, GE Healthcare, USA), each equipped with its own four- or eight-channel phased array breast coil. The routine protocols of our center included the following sequences: axial fast spin echo (FSE) T1-weighted imaging (T1WI), axial fat-suppressed spin echo T2-weighted imaging (T2WI), axial echo-planar diffusion-weighted imaging (DWI), and a sagittal dynamic contrast enhancement (DCE) sequence. Six dynamic sagittal sequences were acquired once before and five times after injection of contrast agent (Gd-DTPA, 0.2 ml/kg body weight, flow rate 2.0 ml/s) at 60 s intervals, immediately followed by an axial delayed contrast enhancement sequence. The relevant parameters for each sequence are listed in Appendix E2.
Image interpretation
Two radiologists, each with 6 and 8 years of experience in breast imaging diagnostics, independently analyzed all MRI manifestations according to the American College of Radiology 2013 Breast Imaging Reporting and Data System (BI-RADS) lexicon. Both observers were blinded to the histopathological information. They assessed the conventional imaging findings of the lesions, including lesion type, tumor size, lesion location, tumor margin, time-intensity curve (TIC) pattern, BPE, AVS, and tumor ADC value. All images were sent to Advantage Workstation (AW 4.6 and AW4.7, GE Healthcare) for further post-processing, including measurement of TIC and ADC values, using Functool and READY View software. Circular ROIs accounting for approximately 75% of each lesion after avoiding areas of hemorrhage or necrosis were drawn and used. TIC patterns were evaluated and categorized into three types (I, II and III): progressive enhancement, plateau and washout patterns. BPE was divided into two categories: extremely minimal (or mild) and moderate (or marked) contrast enhancement. Peritumoral edema was defined as high signal intensity around the tumor on T2WI23AVS positivity was defined as one or more vessels entering the lesion on the T1WI post-enhancement sequence.24. The largest tumor diameter in the image showing the largest lesion was measured and recorded. For the semiquantitative kinetic curve parameters calculated automatically by the Functool software, we measured SI0 (Signal intensity values in the images before contrast administration), SI1 (Signal intensity values in the first images after contrast administration) and SIMax (the maximum signal intensity values in the images after contrast administration). The Early Enhancement Ratio (EER) was calculated as follows: EER = (SI1− S.I.0)/ SI0× 100. The peak enhancement ratio (PER) was calculated as follows: PER = (SIMax− S.I.0)/SI0× 100. Time-to-peak gain (TTP) was also obtained. The reliability of observations was assessed using the intraclass correlation coefficient (ICC). Features with ICCs greater than 0.75 were considered to have satisfactory reproducibility and were reserved for further analysis.
Histopathological examination
The expression levels of estrogen receptor (ER), progesterone receptor (PR), human epidermal growth factor receptor 2 (HER2), and Ki-67, as well as the presence of IMPCs, were pathologically evaluated using hematoxylin and eosin (H&E) and immunohistochemical analysis. ER and PR were considered positive if their expression level was above 1%. HER2 was considered negative if its value was 0 or 1, and positive if its value was 3 or higher. If the HER2 value was 2, fluorescence in situ hybridization (FISH) was required to further confirm HER2 status. High expression of Ki-67 was defined as ≥ 14% and low expression as < 14%. All cases were classified into four immunohistochemical subtypes based on the St. Gallen Consensus Conference 201325: Luminal A, Luminal B, HER2 positive and triple negative.
Segmentation of the volume of interest (VOI) and extraction of radiomic features
Segmentation of all MRI images was performed by a radiologist with 6 years of experience in MRI interpretation. Then, 100 randomly selected cases were assigned to another radiologist with 8 years of experience in MRI interpretation. Both radiologists were unaware of the patients’ clinical data. An example of the segmentation process is shown in Fig. S1. Feature extraction was performed using the Radiomics module in the 3D Slicer software. A total of 851 radiomics features were extracted from each ROI. These features included 107 original features and 744 wavelet-based features (consisting of first-order statistics, gray-level co-occurrence matrix (GLCM), gray-level size zone matrix (GLSZM), gray-level run-length matrix (GLRLM), gray-level dependency matrix (GLDM), neighboring gray-tone difference matrix (NGTDM), and shape-based features). A description of the initial 851 features can be found in Appendix E3.
Radiomic and clinical-radiological feature selection
Feature selection and ML/DL model development were performed on the training cohort, while the validation cohort remained completely independent and invisible until the final model performance was evaluated. Feature standardization (standard deviation) was performed before feature selection. The selection of robust radiomic features involved three steps. First, features with high pairwise correlations at the level of |r| ≥ 0.9 were removed by Pearson correlation analysis to reduce the risk of overfitting. Second, we performed univariate selection using the SelectKBest method for each feature, and features with p-values < 0.05 were retained for further analysis. Third, the least absolute shrinkage and selection operator (LASSO) method was applied to find the optimal log (λ) value by five-fold cross-validation to obtain the robust radiomic features with a non-zero coefficient for differentiating the LVI and non-LVI groups. Independent clinicoradiological characteristics were selected from the variables that were found to be significant in the univariate analysis using multivariate logistic regression analysis.
Model construction and validation
Four different ML classification algorithms (random forest (RF), logistic regression (LR), support vector machine (SVM), and stochastic gradient descent (SGD)) and one DL classification algorithm (multilayer perceptron (MLP)) were used to build models to predict LVI in the training cohort. We optimized the parameters of the different algorithms using five-fold cross-validation. The optimal hyperparameters for each algorithm were determined based on the evaluation results. The relevant hyperparameters are presented in Appendix E4. Prediction models for the radiomic signature (model 1), selected clinical radiological variables (model 2), and integrated features (combination of the two parameters mentioned above, model 3) were built for the training cohort and validated in the validation cohorts.
The performance of the models was evaluated using discrimination metrics including receiver operating characteristic (ROC) curve, area under the ROC curve (AUC), accuracy, sensitivity, specificity, positive predictive value (PPV), and negative predictive value (NPV). The AUCs of the models were compared using the DeLong test.
Statistical analysis
Statistical analyses were performed using MedCalc® Version 20.0.3 (MedCalc Software Ltd, Ostend, Belgium) and R software version 4.2.2 (http://www.Rproject.org). Continuous variables are presented as mean ± standard deviation (SD) and categorical variables as frequencies or percentages, unless otherwise stated. The Kolmogorov-Smirnov test was used to test the normality of data distribution. Continuous variables were compared using the Mann-Whitney test. U test, while dichotomous qualitative variables were assessed using the chi-square test or Fisher’s exact test. Univariate and multivariate logistic regression methods were used to determine the association between clinicopathological and radiological features and LVI. Radiomic feature selection and model development were performed using the Shukun Medical Research Platform (https://medresearch.shukun.net/project; Appendix E5). All tests were two-sided, and a p-value of <0.05 was considered statistically significant.