The economics of AI points to the value of good data – TradingView News
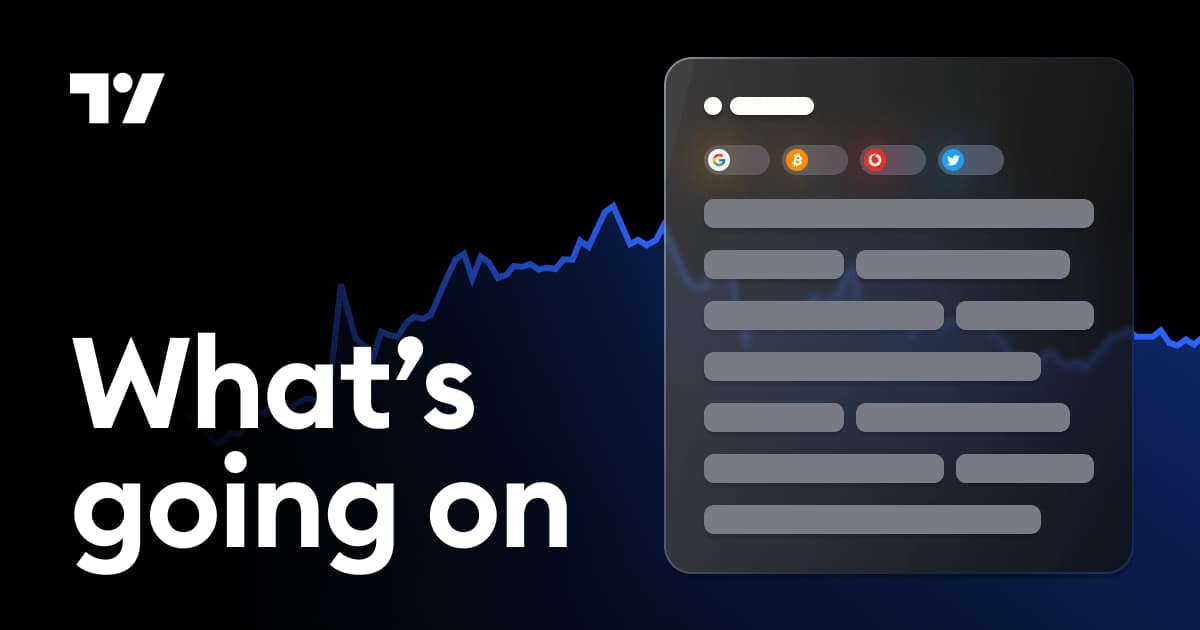
NVIDIA NVDA briefly became the world’s most valuable company last week after shares of the leading supplier of chips and network infrastructure used to train artificial intelligence models nearly tripled since January. But the AI revolution has been anything but a one-way street so far: Most stocks in a number of AI-focused indexes and funds are down this year.
Even for Nvidia’s $3.1 trillion stock, it’s been a wild ride. In the three trading days following its all-time high, it lost more than $400 billion in market value, compared to $360 billion in the week before. Over the past three years, its share price has been five times more volatile than the S&P 500 index.
These huge swings reflect investor uncertainty about the economics of AI. The successes and promises of self-learning computers are clear. How much the technology will cost and who will pay for it is less clear. For investors trying to navigate this treacherous environment, it’s important to start with the technological advances on which the current AI revolution depends.
The stunning applications that have sparked the AI boom seem, at first glance, very different. In March 2016, Google DeepMind’s AlphaGo program wowed the world when it beat all-time star Lee Sedol at the two-person board game. In November 2020, the company’s AlphaFold algorithm cracked one of the life sciences’ greatest challenges by predicting the protein structures that will form novel combinations of amino acids. Two years later, OpenAI seemed to be doing something completely different again when it launched a natural language chatbot that could improvise Shakespeare verses.
Yet all of these milestones rely on the same innovation: a dramatic improvement in the accuracy of computer-based predictive models, as AI pioneer Rich Sutton explained in a 2019 blog post. For decades, researchers have trained computers to play games and solve problems by encoding painstakingly acquired human knowledge, effectively trying to mimic our ability to think logically. But those attempts were ultimately outperformed by a far less complicated approach. Naive learning algorithms consistently proved superior when given enough computing power and enough data. “As we build in our discoveries,” Sutton concluded, “it only becomes more difficult to see how the discovery process can work.”
This lesson is well known. In the 2015 bestseller Superforecasting: The Art and Science of Prediction, Canadian psychologist Philip Tetlock and his co-author Dan Gardner explained that the same agnostic method works for humans too. In prediction tournaments, methodical and open-minded amateurs systematically perform better. Common sense and a willingness to absorb lots of data are more effective than deep domain knowledge and expertise. Today’s groundbreaking AI models essentially automate the super-forecasters’ approach.
This simple recipe – learning algorithms plus computing power plus data – leads to amazing predictive results. It also provides guidance on where the long-term value of AI lies.
Let’s start with the algorithms. Nonprofit research institute Epoch AI estimates that the computing power required to reach a certain performance threshold halved approximately every eight months between 2012 and 2023. That’s the cost savings achieved by recent innovations in neural networks.
But the long-term value of these algorithms is much harder to determine. Digital code is vulnerable to imitation and theft. The pace of future innovation is difficult to predict. The human talent currently sitting in the AI labs of the tech giants could leave at any time.
The second ingredient – raw computing power – is a simpler proposition. According to Epoch AI, it has generated the lion’s share of the performance improvements in AI models. The rising stock market values of the largest cloud computing providers – Alphabet GOOGAmazon
Amazon and Microsoft
MSFT – suggests that stock markets have already priced in many of the gains. But a new manifesto for AI investing by former OpenAI employee Leo Aschenbrenner argues that investors should not be deterred.
Because the performance of the models is closely tied to the amount of chips and power deployed, he urges investors to “trust the trend lines” and “count the OOMs” – a reference to the orders of magnitude by which performance has accelerated year over year – to estimate capital expenditure.
This leads to such enormous demands that even the most optimistic forecasts of the industry are dwarfed. In December, Nvidia competitor AMD AMD Forecasts say the AI chip market will reach $400 billion by 2027. If trend lines are to be believed, AI investments will reach $3 trillion just a year later, while the first $1 trillion data center cluster will open two years later. Add in the OOMs, and it seems that computer hardware, not software, is currently taking over the world.
But there is a flaw in this argument. The first two components of AI – algorithms and computing power – are worthless without the third component: data. And the better the data, the less valuable the computing power becomes.
This fact has been easily overlooked. The most well-known AI applications are general-purpose chatbots trained on huge amounts of unverified text from the internet. They favor quantity over quality and have left processing power to compensate. Morgan Stanley estimated that training OpenAI’s ChatGPT 4 required at least 10,000 graphics chips to process well over 9.5 petabytes of text. This trade-off resulted in remarkably lifelike conversationalists prone to incorrigible hallucinations and increasingly at risk of costly copyright infringement lawsuits.
Specialized applications of AI are less well known, but show where the future lies. Nobel Prize winner Venki Ramakrishnan said Google DeepMind’s AlphaFold model solved “a 50-year-old grand challenge in biology.” Equally remarkable is the fact that it required fewer than 200 graphics chips. This was possible because it was trained on a carefully curated database of 170,000 protein samples. High-quality data therefore not only radically improves the effectiveness of AI models, but also the economics of the technology.
Companies that have useful, specialized data will be the biggest winners from AI. It’s true that highly valued tech giants like Google owner Alphabet and Amazon also dominate some of this space. But far less glamorous – and cheaper – banks, utilities, healthcare providers and retailers are also sitting on AI gold mines.
The real value of the AI revolution lies in data sets, not data centers.

Follow @felixmwmartin on X