From proof of concept to practical implementation

As artificial intelligence (AI) is increasingly used across industries, the transition from proof of concept (POC) to scalable solutions is becoming increasingly important. According to a recent study, up to 90 percent of AI and generative AI (GenAI) projects are stuck in the POC phase and do not move into production.
At Thoughtworks, we see a new kind of urgency emerging in 2024. Leadership teams are demanding real results from their early attempts at AI. But this requires organizations to recognize that AI is not a standalone tool and is not as simple as plug-and-play.
AI success depends on companies pursuing an iterative AI strategy guided by constant experimentation, robust engineering practices, and clear guardrails. This approach could require rethinking how a company works.
Laying the foundations for AI implementation
Companies need to put some fundamental building blocks in place before they can benefit from the new breakthroughs in AI that seem to emerge every day. One of these is a solid data strategy that ensures a baseline level of relevant, credible and actionable data is available to feed into AI models. Without this foundation, an AI solution can lead the company to make bad decisions faster.
It is also important to deploy tools like GenAI with a basic understanding of what “good” means for the outcome leaders want to achieve. While these tools can be controlled, they cannot be trusted to work without oversight or to verify the quality of their own outputs. Providing tools and processes to continuously monitor and evaluate the outputs of AI systems is part of responsible technology practice and essential to avoid unintended consequences.
Once these parameters are established, Thoughtworks encourages organizations to test AI against potential use cases in their operations. As with all innovations, it can be difficult to understand the full potential or breadth of applications until the technology is firmly in use.
High-quality labelled data and data access
Another common challenge that prevents companies from deploying their models in production is the lack of transparency around complex AI models. This “ambiguity” makes it difficult to assess accuracy and suitability for specific requirements. Thoughtworks helps companies address this problem by providing tools and expertise to reliably evaluate Large Language Models (LLMs).
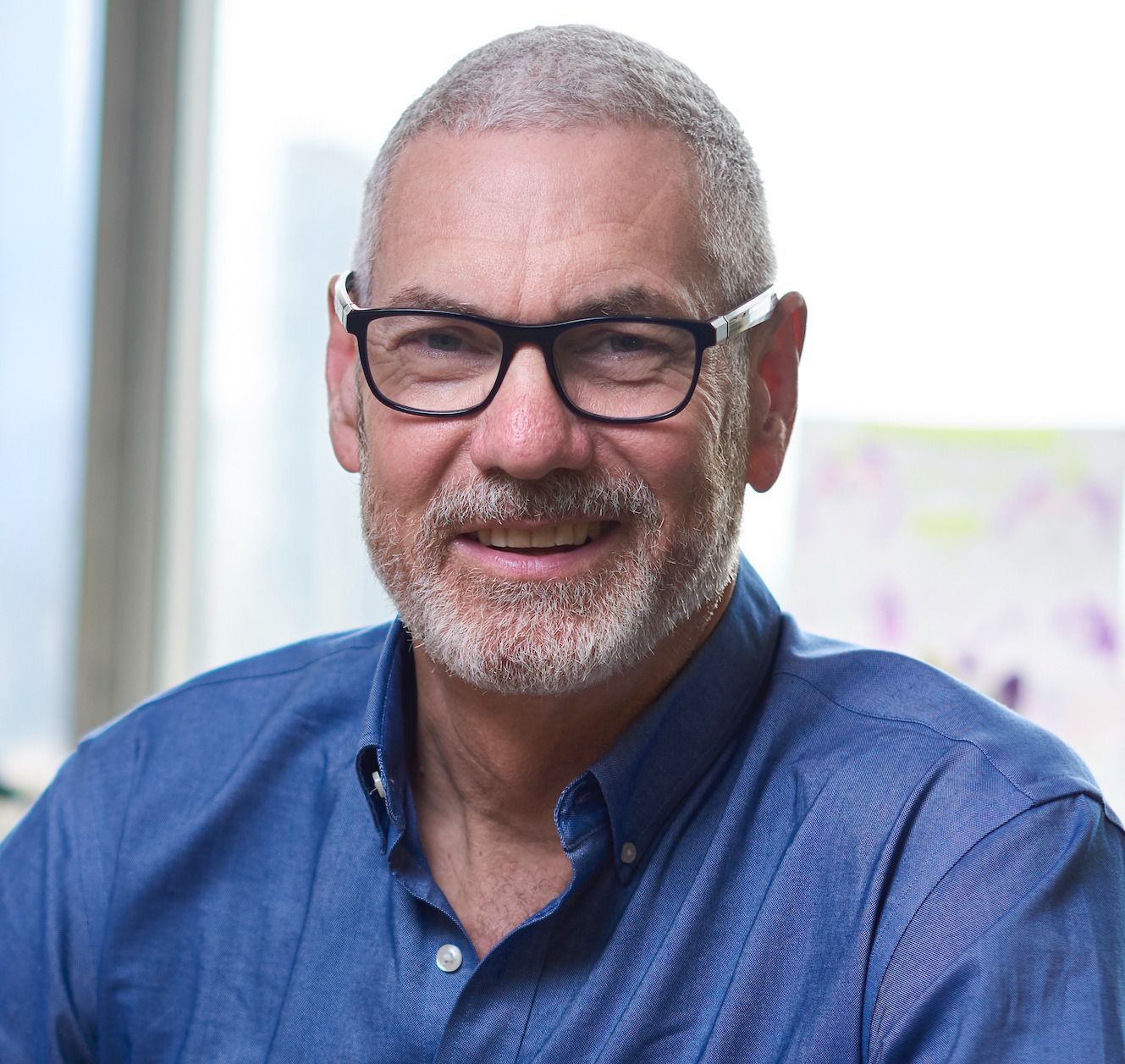
By offering accelerators for tasks such as text classification and data labeling, Thoughtworks’ pre-built solutions streamline the development process and encourage companies to move beyond the proof-of-concept (POC) phase and achieve faster results with their AI projects.
By gaining greater confidence in the use of AI models, organizations can address the opaque nature of LLMs and make more informed decisions. Executives can correctly answer questions such as “How do I know if the LLM results are accurate?” or “Which model/approach is best for my use case?”
Aside from data labeling, it is important that AI POCs reflect and adhere to your organization’s privacy and security policies. Incorporating existing access controls into the LLM’s behavior not only increases security but can also reduce training costs.
For example, when integrating an LLM into a data platform, context and model output should take into account the user’s role and access rights. This ensures that users only access data they have permission to view, increasing overall system security.
Effective prompt
Currently, developing effective prompts relies heavily on trial and error, making it difficult to scale and maintain GenAI solutions. These prompts, which drive AI responses, can become ineffective as models evolve. Thoughtworks’ solution addresses this problem by building tools that optimize prompts for specific models. This not only simplifies production maintenance of GenAI applications, but also enables better portability between models – ensuring that organizations can leverage the model that best fits their needs without having to start from scratch with prompt design.
yuu Rewards Club: a case study on rapidly scaling AI
yuu Rewards Club, Singapore’s leading coalition loyalty platform, is an example of how AI can be used to scale rapidly. It integrates top brands across retail, hospitality, entertainment, banking and more, offering a hyper-personalized mobile experience and a unified currency for maximum rewards.
Equipped with advanced AI and ML capabilities and a robust partner ecosystem, the platform is revolutionizing traditional loyalty programs and offering consumers new shopping experiences, such as convenient redemption of offers from multiple brands through a single app, as well as personalized offers and rewards.
Developed by minden.ai, a technology company founded by Temasek, in collaboration with Thoughtworks, the platform skyrocketed to become the number one app in both major app stores within a month, and gained over a million members in just 100 days.
This is a great example of how user-centered design, agile development, and a focus on scalability can help achieve rapid growth with AI-powered platforms.
South Asian Bank’s GenAI chatbot revolutionizes customer service
Thoughtworks worked with a leading South Asian bank to address a common challenge: scattered data was impacting the customer experience. Data was spread across different sources, making it difficult for product managers to access customer information efficiently.
Using GenAI, the team analyzed the datasets, identified key pain points, and developed a production-ready GenAI-based chatbot. They also created a reusable framework that could be adapted to any fine-tuned language model to ensure scalability.
The GenAI agent proved to be a game-changer, significantly improving customer service capabilities and providing users with a more streamlined conversational experience.
Responsible AI
In both cases described above, the rapid transition from POC to volume production was facilitated by strong executive support. Such organization-wide buy-ins are complemented by dynamic GenAI strategies that can keep pace with the rapidly evolving market and user demands.
Organizations should also establish a responsible AI framework that addresses critical issues such as privacy, security, and compliance with laws and regulations. As AI and its capabilities continue to evolve, safeguards are essential to ensure its ethical and responsible use. For example, we have a comprehensive Responsible Tech Playbook, in collaboration with the United NationsIn addition to AI, considerations regarding sustainability, data protection and accessibility are also taken into account.
For companies looking to make the most of AI, true success lies not just in automating routine tasks, but in enhancing human capabilities and increasing the impact of individual contributions within the organization.
The author is the APAC Chief Technology Officer of Thoughtworks.
To learn about real successes and challenges in navigating this AI transition, join the webinar on July 25 with experts from AWS, PEXA and Thoughtworks. Register now.