The advantages, use cases and the measurement value
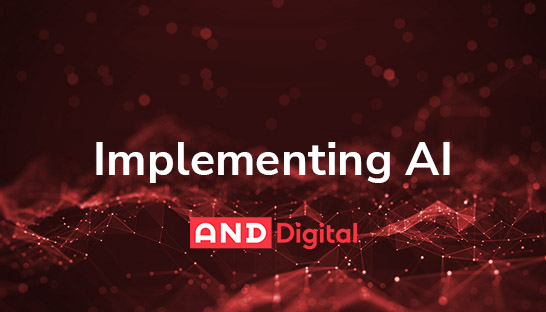
AI is presented by many as a panacea and progress in all possible areas. But really benefiting from AI is easier said than done. Experts from AND Digital explain how the right use cases can be identified and which metrics should be used to ensure a positive return on investment.
Measurable value should always be the end goal of any technology adoption, and artificial intelligence (AI) is no exception. Ultimately, the key to AI success is ensuring that the technology creates value for the business, stakeholders, and/or society as a whole.
If implemented successfully, the benefits of AI can be significant or even game-changing. According to a recent study, the technology could add trillions of dollars in value to economies and industries every year.
What is AI good at?
An important starting point on the AI journey is to identify where AI really adds value. In fact, there are some areas where AI really excels – and can outperform a human – and others where human intervention is definitely still required. To quickly identify value, it is wise to focus on the strengths of AI and consider how these can be used in an organization.
- AI is great at tedious tasks like data tagging, metadata tagging, and pattern recognition. Due to the tedious nature of these tasks, there is a higher risk of errors when performed by humans, and humans also take much longer
- AI is great at processing large amounts of data and delivering insights quickly, and it can free up employees to focus on higher-value activities.
- AI is great at identifying patterns, and when applied in the right context, it can lead to insights and opportunities that might not have been possible otherwise.
Consider the business challenges
Once an understanding of what AI does well is gained, leaders can consider the challenges facing their organization. A strong use case is based on matching technological capabilities with real organizational needs. After all, leaders don’t want to end up with a “solution in search of a problem.”
So how can leaders identify the right use cases for AI? Five key factors:
(1) Identify the right problem
The first step is to understand what problems or opportunities exist in the organization that could be addressed by AI. This could be improvements in customer service, recommendation engines or machine learning models that predict outcomes or simulate real-world scenarios.
(2) Feasibility and impact
Once a potential use case is identified, it is important to evaluate its feasibility and potential impact. This requires asking questions such as: Can the problem be clearly defined? Is relevant data available to train AI models? What is the expected ROI? This helps prioritize the use cases that will provide the most value.
(3) Integration and scalability
Consider how the proposed AI solution will integrate with existing systems. It’s important that the solution is scalable and adapts as the business grows and changes. If an AI tool doesn’t integrate well with existing processes – or is too rigid – it may end up causing more problems than it solves.
(4) Ethical and legal considerations
AI implementations must also be thoroughly assessed for ethical and legal implications, particularly with regard to data usage and privacy. Compliance with regulations such as GDPR is critical to maintaining trust and integrity when using AI.
(5) Continuous learning and adaptation
Finally, the successful implementation of AI is not a one-time project, but an ongoing process. AI systems need to be continuously trained and updated to adapt to new data and changing business environments. Companies must be willing to invest in the continuous training and development of their AI systems and ensure that their employees have the skills needed to add value.
Measuring the ROI of AI use cases
While the business benefits of AI can vary depending on the use case, there are several metrics that can be used to estimate and monitor the benefits of AI initiatives in advance:
AI metrics before implementation:
- Estimated costs of developing AI models – including the costs of data labeling, model training, testing, and more
- Estimated ongoing maintenance costs – the expected cost of updating an AI model over time as more data is collected
- Time spent on current manual processes – Track the hours specific roles spend on tasks that can be automated
- Error/rework rates of current processes – Track errors or rework caused by manual tasks such as data entry
- Customer satisfaction with current processes – conduct surveys to understand the pain points customers experience with current processes
AI metrics after implementation:
- Actual costs of developing and maintaining AI models – compare expenses with estimates to determine variances
- Time savings through automated processes – track the hours saved by different professional groups by reducing manual tasks
- Increased productivity – Track the number of claims/orders processed and tasks completed by roles per unit/time.
- Reduced error rate – Track errors in automated tasks compared to manual execution either as a percentage or as a number
- Improved first contact resolution – track whether AI helps resolve queries on first contact versus multiple contacts
- Reduced support costs – track the reduction in calls/emails to support teams in terms of both volume and cost savings.
- Increased customer satisfaction – Repeated surveys to measure any improvements in the experience with the new automated processes
- Growth in relevant KPIs – Track metrics such as order value, customer lifetime value and churn rates over time, aligned with business goals.
Increased value through AI
A summary of the key things leaders need to know about building an effective AI use case to create demonstrable value for the business:
First, start by clearly defining a specific problem statement and a desired, quantifiable outcome. This provides a “north star” to guide the project. Then focus on solving strategic business problems in the long term, not just implementing technology for its own sake in the short term. The focus should always be on business value, not “shiny objects.”
Finally, think carefully about relevant quantitative and qualitative ways to measure long-term ROI and business value.