Association between panimmune inflammation score at admission and short-term mortality in septic patients: a retrospective cohort study
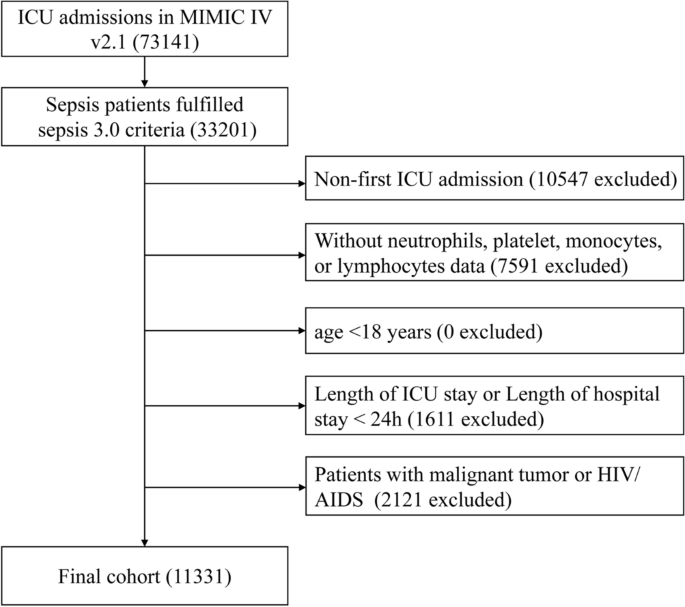
Data sources
This study was based on a publicly available intensive care database called the Multiparameter Intelligent Monitoring in Intensive Care (MIMIC) IV database (version 2.1). MIMIC IV contains anonymized intensive care patient data from Beth Israel Deaconess Medical Center between 2008 and 2019.12. The Institutional Review Boards of Beth Israel Deaconess Medical Center and Massachusetts Institute of Technology approved this database. This study was published according to the guidelines for strengthening the reporting of observational studies in epidemiology.13. In addition, this study was exempt from our Institutional Review Board (IRB) approval because it was based on a public, anonymized database with pre-existing IRB approval. Clinical data were extracted by HB Xu (35,959,043), who gained access to the database after successfully completing an online training course.
Participant
Adult septic patients were initially enrolled according to Sepsis 3.0 criteria. Patients were excluded if they met any of the following criteria: (a) not first-time ICU admission; (b) no data on neutrophil, platelet, monocyte, or lymphocyte counts on the first day of ICU admission; (c) age < 18 years; (d) duration of ICU or hospital stay less than 24 hours; (e) diagnosed malignancy (including hematologic neoplasms), human immunodeficiency virus (HIV) infection, or acquired immunodeficiency syndrome (AIDS).
Data extraction
Data on demographic parameters, comorbidities, vital signs, laboratory parameters, scoring systems, and supportive therapies were extracted from MIMIC IV using PostgreSQL software (version 9.6). Demographic parameters included age, ethnicity, and gender. Comorbidities included hypertension, diabetes mellitus, myocardial infarction, heart failure, chronic lung disease, severe liver disease, chronic kidney disease, and cerebrovascular disease. Vital signs included temperature, respiratory rate, heart rate, mean arterial pressure (MAP), and pulse oxygen saturation (SpO2). Laboratory parameters included white blood cells (WBC), hemoglobin, erythrocyte distribution width (RDW), glucose, serum creatinine, urea-bound nitrogen (BUN), anion gap, bicarbonate, sodium, potassium, prothrombin time, partial thromboplastin time, international normalized ratio (INR), lactate, neutrophils, platelets, monocytes, and lymphocytes. Scoring systems included Sequential Organ Failure Assessment (SOFA) score and Simplified Acute Physiology Score II (SAPS II). Supportive therapies included the use of renal replacement therapy (RRT), mechanical ventilation, and vasoactive agents during the first day after ICU admission. Comorbidities were diagnosed according to International Classification of Diseases (ICD)-9 and ICD-10 codes. Due to lack of relevant information, it was difficult to identify patients with septic shock in this database. Therefore, data on the use of vasoactive agents were extracted for subgroup analysis. If a laboratory parameter was tested multiple times during the 24-hour ICU stay, the first value was extracted for analysis. The PIV index was calculated using the formula “neutrophil count (k/ul) × monocyte count (k/ul) × platelet count (k/ul)/lymphocyte count (k/ul)”.6.
Results
The primary endpoint was all-cause mortality within 28 days of ICU admission, and the secondary endpoint was all-cause mortality within 90 days.
Dealing with missing data and outliers
Missing data are common in the MIMIC IV database. The proportion of missing values of the extracted variables was less than 5%, except for lactate, which had a high proportion of missing values (24.6%). Details of missing values can be found in the Supplementary Materials (Table S1). Since serum lactate is an important variable in sepsis, it was treated as a dummy variable and assigned a category to indicate the missing status. The remaining variables with missing values were replaced with the respective mean or median value when appropriate. In addition, variables with abnormal values were adjusted using the winsor2 command with replace cuts (1.99). STATA software (version 14) was used to manage the missing data and outliers.
Statistical analysis
Due to its skewed distribution, PIV was log2 transformed before analysis. Continuous variables were expressed as mean ± standard deviation (SD) or median (interquartile range) and compared using univariate analysis of variance or Kruskal-Wallis test. Categorical data were presented as numbers (percentages) and analyzed using chi-square or Fisher exact tests. Survival curves were estimated using Kaplan-Meier survival analysis and compared using log-rank test. The potential association between log2 PIV and the risk of 28-day and 90-day all-cause mortality was assessed using unadjusted and multivariable-adjusted Cox proportional hazards models. In multivariable analysis, adjusted covariates were selected based on clinical relevance and a change in effect estimate of more than 10%.14,15. The assumption of multicollinearity was also assessed and a variance inflation factor (VIF) greater than 5 indicates the presence of multicollinearity between independent variables. Model 1, unadjusted; Model 2, adjusted for age, ethnicity and sex; Model 3, adjusted for age, ethnicity, sex, SOFA, SAPS II, mechanical ventilation, renal replacement therapy, vasoactive agents, myocardial infarction, heart failure, hypertension, diabetes mellitus, chronic lung disease, liver disease, chronic kidney disease, cerebrovascular disease, temperature, respiratory rate, MAP, SPO2, WBC, hemoglobin, BUN, anion gap, bicarbonate, lactate, RDW, glucose, creatinine, INR. The shape between log2-PIV and survival endpoints was examined using restricted cubic spline analysis. If a nonlinear association was found, a two-part regression model was performed to elucidate the nonlinearity. Additionally, we examined the association between PIV and the risk of 28- and 90-day mortality in several subgroups, including age (< 65 and ≥ 65 years), sex, race, myocardial infarction, heart failure, hypertension, diabetes mellitus, chronic lung disease, severe liver disease, chronic kidney disease, cerebrovascular disease, renal replacement therapy, ventilator use, use of vasoactive medications, SOFA (< 6 and ≥ 6), and SAPS II (< 40 and ≥ 40). Finally, we performed two sensitivity analyses to verify the results. First, patients with missing values were excluded. Second, patients with autoimmune diseases, including systemic lupus erythematosus, systemic sclerosis, ulcerative colitis, rheumatoid arthritis, ankylosing spondylitis, Crohn's disease, multiple sclerosis, myasthenia gravis, and polymyositis, were excluded due to their potential impact on WBC count.16. Data analyses were performed using R 4.2.1 (http://www.R-project.org) and Free Statistics software (version 1.7.1, Free Clinical Medical Technology Co., Ltd, Beijing, China). A two-sided P A value < 0.05 was considered statistically significant.
Statement from the Institutional Review Board
Use of the MIMIC database was approved by the Institutional Review Board of the Massachusetts Institute of Technology and Beth Israel Deaconess Medical Center.